What Is Black Box AI? How Does It Work?
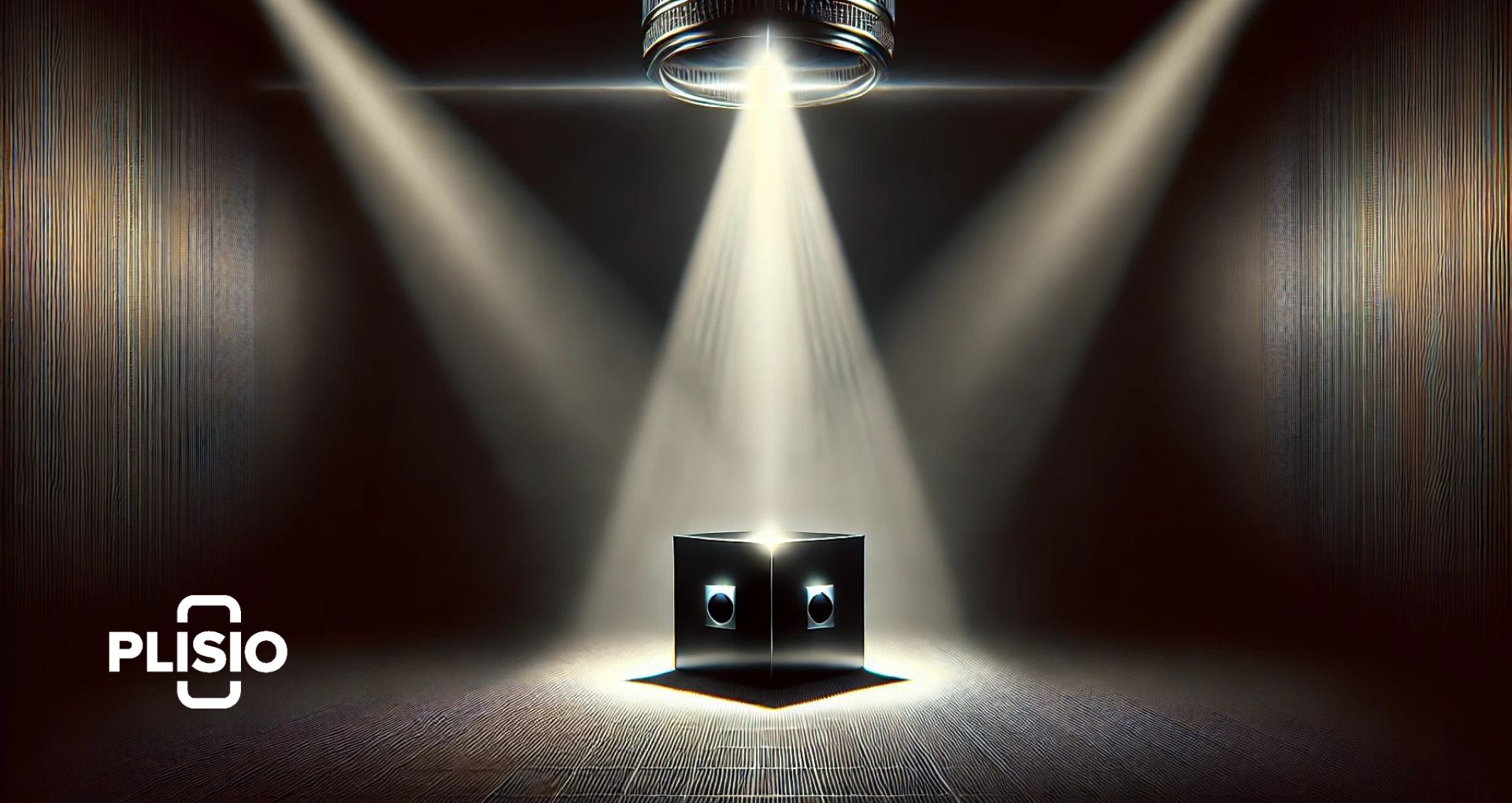
In recent years, Black Box AI has gained prominence due to its impressive ability to handle complex data and provide accurate predictions. However, its inner workings remain opaque, making it difficult for users to understand how decisions are made. This lack of transparency raises concerns about trust, ethics, and accountability, especially in high-stakes industries like healthcare, finance, and law enforcement. As AI technology continues to advance, efforts are being made to improve the interpretability of these models through Explainable AI (XAI) and regulatory measures, aiming to strike a balance between AI's power and the need for transparency. This article delves into the challenges and implications of black box AI, exploring its applications, ethical concerns, and the future of AI in an increasingly regulated and transparent landscape.
What Is BlackBox AI?
Black box AI refers to artificial intelligence systems where the internal processes remain hidden from users, making it difficult to understand how decisions are made. In these systems, complex algorithms, often utilizing deep neural networks, process data in a way that mimics human brain function—dispersing inputs across numerous artificial neurons. The resulting complexity makes it challenging, if not impossible, for humans to grasp the rationale behind the AI's decisions. This creates a situation where the outcome is clear, but the steps leading to that outcome are obscure.
The term "black box" dates back to the 1940s when it was first used in the aerospace industry to describe flight recorders. Over time, as AI evolved, particularly with the development of intricate machine learning models, the term found its place in the realm of artificial intelligence. Today, black box AI systems are becoming increasingly common across industries, despite their opaque nature.
In contrast, white box AI offers transparency by making its decision-making processes explainable. Users can understand each step the system takes, allowing for greater accountability and trust. To illustrate the difference, think of black box AI as using a secret spice mix while cooking—you get a delicious result but have no idea why. On the other hand, white box AI is like following a precise recipe where every ingredient and step is known and understood.
How Does Black Box Machine Learning Work?
Black box machine learning models, particularly those based on deep learning, are designed to process large volumes of data and learn complex patterns autonomously. These models are called "black boxes" because their internal workings—how they analyze inputs and generate outputs—are hidden from the user and often too complex to fully understand. The development of these models typically follows a sequence of steps aimed at enabling the AI to make accurate predictions based on real-world data.
Here’s an overview of the process:
- Data Ingestion and Pattern Recognition: The model is fed millions of data points, which could include anything from images to text or financial records. Using sophisticated algorithms, the AI examines this extensive dataset to identify underlying patterns. It experiments through trial and error, constantly adjusting its internal parameters until it can reliably predict outcomes.
- Training Through Feedback: During the training phase, the model learns by processing vast amounts of labeled data (input-output pairs). For example, it might be tasked with predicting whether a financial transaction is fraudulent. The model tweaks its internal parameters, based on feedback, to minimize the gap between its predicted outcomes and the actual labels provided in the data.
- Prediction and Scaling: Once the model has been trained, it begins to make predictions based on new, unseen data. In real-world applications, such as fraud detection or medical diagnostics, the model continuously refines its predictions as more data is introduced. Over time, the AI system scales its knowledge and adapts to changing inputs, improving its performance.
However, one of the main challenges of black box machine learning models is their lack of transparency. Since the algorithms independently adjust themselves and develop their own pathways, it becomes incredibly difficult, even for experienced data scientists, to trace how the model arrives at a specific decision. This opacity creates issues of trust and accountability, particularly in critical applications like healthcare or finance, where understanding the logic behind a decision is essential.
Although techniques such as sensitivity analysis and feature visualization have been developed to give insights into how the model is functioning internally, these methods provide only limited visibility. These techniques might highlight which features (or data inputs) the model considered most important, but the exact decision-making process remains elusive.
The Push for Explainability
As machine learning systems become increasingly integrated into everyday technologies, the demand for explainable AI (XAI) has surged. Regulators, businesses, and even users want more transparency from AI systems, especially in scenarios where automated decisions impact human lives. For instance, the European Union has been pushing for regulations that require AI models, particularly those used in sensitive domains, to be interpretable and accountable.
Techniques like LIME (Local Interpretable Model-agnostic Explanations) and SHAP (SHapley Additive exPlanations) are being developed to help bridge the gap between complex black box models and the need for understandable outputs. These approaches offer a window into how individual predictions are made, offering explanations that non-experts can grasp.
However, the balancing act between maintaining the accuracy and power of deep learning models while increasing their explainability remains one of the most pressing challenges in the AI field today.
What Are The Implications of Black Box AI?
Black box AI, while powerful, presents several critical challenges and risks that organizations need to consider carefully before adopting these systems. As deep learning models, which often operate as black boxes, continue to gain popularity, their lack of transparency, potential for bias, and vulnerability to security flaws make them both valuable and dangerous tools.
1. Lack of Transparency and Accountability
One of the most significant concerns about black box AI is the opacity surrounding its decision-making process. While these models can generate accurate results, even their developers often don't fully understand how they arrive at their conclusions. This lack of visibility makes it difficult to audit or explain the AI's decisions, which is particularly problematic in high-stakes industries like healthcare, finance, and criminal justice. In these fields, an AI's opaque decision can directly impact people's lives, making it essential to ensure that the models used are transparent and accountable.
The emergence of Explainable AI (XAI) aims to address these concerns by developing systems that offer more insight into how decisions are made. Despite these efforts, many black box AI models remain challenging to interpret, raising ethical and practical concerns for businesses and regulators alike.
2. AI Bias and Ethical Implications
Another significant issue with black box AI is its susceptibility to bias. Bias can creep into the system through the training data or the unconscious prejudices of developers. For example, if an AI model used for recruitment is trained on historical data where most employees were male, it may develop a bias against female applicants, even though such bias is unintended. When organizations deploy black box models without being able to identify or address these biases, they risk reputational damage, legal action, and the ethical dilemma of perpetuating discrimination.
In sectors such as law enforcement, the consequences of biased AI could be even more severe, as biased decisions might lead to unfair treatment of certain groups. These risks highlight the importance of incorporating fairness and ethics into AI development, as well as the need for ongoing monitoring and transparency.
3. Accuracy Validation
The lack of transparency in black box AI also makes it difficult to validate the accuracy of the model's predictions. Since it's unclear how the AI arrives at its conclusions, organizations cannot easily test or verify whether the results are reliable. This is particularly troubling in fields like finance or medicine, where mistakes could have severe financial or health-related consequences. If an AI model produces inaccurate results, it may take significant time to detect and correct, leading to flawed decisions and potential damage.
4. Security Vulnerabilities
Black box AI models are also susceptible to attacks from malicious actors. Because the model's internal workings are hidden, threat actors can exploit vulnerabilities in the system, manipulating input data to skew the results. For instance, in a security setting, an attacker might alter data to cause the AI to make incorrect judgments, leading to security breaches or other dangerous outcomes.
Additionally, black box models often require large datasets for training, which increases the risk of data exposure. When sensitive information is involved, such as in healthcare or financial services, ensuring the privacy and security of the data becomes even more critical. Another concern arises when third-party vendors are involved—without transparency, businesses may be unaware that their data is being transferred to external entities, which could introduce further security risks.
5. Lack of Flexibility
Finally, black box AI models are not always flexible. If the model needs to adapt to new data or adjust its internal parameters for different scenarios, it can be challenging and time-consuming to implement those changes. In fields that require regular updates to decision-making processes, this lack of flexibility can be a significant disadvantage, further limiting the usefulness of black box AI in sensitive or rapidly changing environments.
When Should BlackBox AI Be Used?
While black box AI models come with certain challenges, they also offer significant advantages in various high-stakes applications. These systems excel in complex scenarios, providing higher accuracy, efficiency, and automation that can outweigh the drawbacks in certain contexts. Below are some key instances when black box AI can be highly beneficial, as well as examples of its real-world applications.
1. Higher Accuracy in Complex Data Analysis
Black box AI models are known for their high prediction accuracy, particularly in fields like computer vision and natural language processing (NLP). These models can uncover intricate patterns in massive datasets that are difficult for humans to detect, allowing them to make better predictions. For example, in image recognition tasks, black box AI can analyze and categorize visual data with precision far beyond that of traditional models.
However, the same complexity that allows for higher accuracy also makes these models less transparent. The more layers and parameters a model has, the harder it becomes to explain its decision-making process. This trade-off between accuracy and interpretability is one of the most significant challenges with black box AI.
2. Rapid Decision-Making and Automation
Another key advantage of black box AI is its ability to process and analyze data quickly, making rapid conclusions based on predefined rules or models. This speed is critical in applications like stock trading, where decisions need to be made in fractions of a second. Moreover, black box models can automate complex decision-making processes, freeing up human resources and boosting efficiency in industries where time is a key factor.
For example, in self-driving cars, black box AI processes sensor data in real-time to make instantaneous decisions—like adjusting speed or avoiding obstacles—without requiring human input. However, this also raises concerns about the safety and reliability of such models, as accidents involving autonomous vehicles have sparked debates over accountability and trust.
3. Efficiency with Minimal Computational Resources
Despite their complexity, black box models can sometimes be optimized to run efficiently without requiring extensive computational resources. For instance, in some machine learning tasks, once a black box model is trained, it can make predictions with relatively low computing power, making it ideal for applications where large-scale, fast decision-making is essential but hardware resources are limited.
Real-World Applications of Black Box AI
Automotive Industry
One of the most prominent examples of black box AI is in the autonomous vehicle industry. Self-driving cars rely on AI systems to interpret sensor data and make decisions about navigation, obstacle avoidance, and speed adjustments. The AI within these systems operates as a black box—analyzing millions of data points without human oversight or clear insight into its internal workings. While this technology has the potential to revolutionize transportation, it has also raised concerns due to its role in accidents. Many worry that safety risks associated with black box AI in self-driving cars could outweigh the benefits, especially since it’s difficult to trace the exact cause of malfunctions.
Manufacturing
In the manufacturing sector, AI-driven robots and machines have been automating production lines for years. Today, black box AI is used for predictive maintenance, where deep learning models analyze equipment sensor data to predict machine failures and prevent costly downtime. However, if the AI model makes an incorrect prediction, it can lead to product defects or safety hazards, and the lack of transparency makes it difficult to identify the cause of the failure.
Financial Services
Black box AI is also extensively used in the financial industry. Trading algorithms powered by AI can analyze massive datasets on market trends and execute trades at lightning speed. Additionally, AI models help assess credit risk by analyzing consumer financial histories. Despite their efficiency, black box AI models have been flagged by regulators as a potential vulnerability due to the risk of producing errors or biases that may go undetected.
For example, U.S. financial regulators have raised alarms over the potential for AI models to generate misleading results, which could destabilize financial markets. Concerns also revolve around data privacy and the risk that hackers could exploit the opacity of these models to gain unauthorized access to sensitive financial information.
Healthcare
In the healthcare industry, black box AI is used to assist doctors in diagnosing diseases and developing treatment plans. For example, AI models can analyze medical images or patient histories to detect early signs of conditions like cancer or heart disease. While these tools have the potential to save lives, they also raise ethical concerns. If the AI model is biased or flawed, it could lead to misdiagnoses or inappropriate treatment recommendations, endangering patients' health.
The opacity of black box AI in healthcare makes it difficult to pinpoint the root cause of mistakes, which can erode trust in these systems. As AI becomes more integrated into patient care, there is a growing push for models that are not only accurate but also explainable and transparent.
What Is Responsible AI?
Responsible AI (RAI) refers to the development and deployment of artificial intelligence in a manner that aligns with ethical values and societal norms. It ensures that AI systems are designed, implemented, and managed in ways that are morally upstanding, socially responsible, and legally accountable. The goal of RAI is to mitigate potential negative impacts—such as financial losses, reputational harm, or ethical violations—by adhering to a set of core principles and best practices.
As AI technologies become more integrated into daily life, from healthcare and finance to transportation and customer service, the importance of responsible AI is growing. Ensuring that these systems operate fairly and transparently not only protects consumers but also builds trust and reduces risks for organizations using AI.
Key Principles of Responsible AI
Responsible AI practices are guided by the following key principles, which help ensure that AI is developed and used in a way that benefits society as a whole:
1. Fairness
One of the most critical aspects of responsible AI is ensuring fairness in the way the system treats individuals and demographic groups. AI systems must avoid perpetuating or amplifying existing biases in society. For example, AI models used in hiring, lending, or law enforcement should be carefully monitored to ensure they do not unfairly disadvantage certain groups based on factors like race, gender, or socioeconomic status.
To achieve fairness, RAI focuses on building models that are trained on diverse datasets, free from bias, and tested regularly to ensure their outputs remain equitable.
2. Transparency
Transparency is another cornerstone of responsible AI. Transparency means that AI systems should be understandable and explainable to all stakeholders, from end-users to regulators. This requires AI developers to clearly communicate how their models are built, how they function, and what data they rely on for training.
Additionally, organizations must disclose how they collect, store, and use data. This openness helps build trust with users and ensures that data privacy regulations, such as the GDPR (General Data Protection Regulation) in Europe, are met. Explainability is particularly crucial when AI systems are used in sensitive areas like healthcare, legal decisions, or credit scoring, where transparency is directly linked to ethical responsibility.
3. Accountability
Accountability ensures that the organizations and individuals responsible for creating, deploying, and managing AI systems are held accountable for the decisions made by the technology. This includes not only the developers of the AI system but also those who implement it in real-world applications.
Accountability frameworks ensure that when AI makes a mistake—such as providing a biased recommendation in a hiring process or making an incorrect medical diagnosis—there is a clear path to correcting the issue and holding the appropriate parties responsible.
4. Ongoing Development and Monitoring
Responsible AI requires continuous development and monitoring. AI systems should not be static; they must be regularly updated and monitored to ensure they continue to align with ethical standards and societal expectations. This is especially important because the real-world data that AI models process is constantly evolving, and so too must the models to avoid outdated or harmful outputs.
Monitoring also helps identify and mitigate any emerging biases or vulnerabilities in the system. For example, in cybersecurity applications, ongoing vigilance is crucial to ensure AI systems remain secure against new types of attacks.
5. Human Oversight
While AI can automate complex tasks, it is critical that AI systems are designed with mechanisms for human oversight. Human involvement is essential in high-stakes applications, such as healthcare, autonomous vehicles, or criminal justice, where AI decisions can have life-altering consequences.
Allowing humans to intervene, review, and even override AI decisions helps ensure that the technology remains aligned with human values and ethics. This principle also helps ensure that AI does not operate unchecked, particularly in situations where moral and ethical judgment is required.
Black Box AI vs. White Box AI
Black box AI and white box AI represent two fundamentally different approaches to developing artificial intelligence systems. The choice between these approaches depends on the specific goals, applications, and requirements for transparency in each use case.
Black Box AI: Power Without Transparency
Black box AI systems are characterized by their opacity—while the input and output are visible, the internal decision-making process remains hidden or too complex to fully understand. These models are commonly used in deep learning, especially in neural networks, where large datasets are processed, and the model adjusts its internal parameters to make highly accurate predictions. However, understanding exactly how these predictions are made is challenging.
Common Use Cases:
- Image and Speech Recognition: Black box AI excels in areas like facial recognition and natural language processing (NLP), where the primary goal is achieving accuracy rather than transparency. For instance, deep neural networks used in voice assistants or image classification systems can deliver high performance, but the inner workings of these models are difficult to interpret.
- Autonomous Vehicles: Self-driving car technology often leverages black box AI, where quick, complex decision-making is crucial for navigating real-world environments in real time.
While black box models tend to be more accurate and efficient than their white box counterparts, they pose challenges in terms of trust and accountability. Since it’s difficult to understand how they arrive at specific conclusions, these models can be problematic in sectors that require high levels of transparency, such as healthcare or finance.
Key Features of Black Box AI:
- Higher accuracy and performance, particularly in complex, data-intensive tasks.
- Difficult to interpret or explain, making it harder to debug or audit.
- Common models include boosting algorithms and random forests, which are highly nonlinear and not easily explainable.
White Box AI: Transparency and Accountability
In contrast, white box AI systems are designed to be transparent and interpretable. Users and developers can examine the algorithm’s inner workings, understanding how variables and data influence the model's decision-making process. This makes white box AI especially valuable in areas where explainability is crucial, such as medical diagnosis or financial risk assessments.
Common Use Cases:
- Healthcare: White box AI is used to assist in medical diagnoses, where doctors and patients need to understand how the system arrived at a particular recommendation. This transparency is essential for building trust and ensuring that the AI's decisions are based on sound logic.
- Financial Analysis: In financial models, transparency is critical for compliance with regulations. White box AI allows analysts to see how risk factors are weighted, ensuring that decisions regarding loans or investments can be easily explained and justified.
Because of its transparency, white box AI is easier to debug, troubleshoot, and improve. Developers can identify and fix issues more efficiently, which is particularly important in applications that involve high stakes or regulatory scrutiny.
Key Features of White Box AI:
- Interpretability: Users can understand how decisions are made, making it easier to ensure fairness and accountability.
- Easier to troubleshoot: Problems can be quickly identified and corrected, thanks to the transparent nature of the system.
- Common models include linear regression, decision trees, and regression trees, which are straightforward and interpretable.
The Trade-Off Between Accuracy and Transparency
One of the key trade-offs between black box and white box AI lies in the balance between accuracy and interpretability. Black box models are often more powerful and capable of handling complex data structures, but they lack transparency. On the other hand, white box models prioritize transparency, making them easier to understand but sometimes sacrificing predictive power.
For instance, neural networks—which are typically considered black box systems—are gaining popularity because of their high accuracy in tasks like image classification and NLP. However, organizations in fields like healthcare and finance are increasingly looking for solutions that combine the accuracy of black box models with the accountability of white box systems, leading to growing interest in hybrid models or explainable AI (XAI).
Black Box AI Solutions
When considering AI decision-making systems, one of the critical questions is whether these systems are as transparent as they need to be. If the answer is no, it's essential to explore solutions to improve the transparency and interpretability of AI algorithms. One of the leading approaches to achieving this is through Explainable AI (XAI).
Explainable AI (XAI)
Explainable AI (XAI) is a specialized branch of AI focused on making the decisions of AI algorithms more understandable to humans. XAI ensures that AI models are not only accurate but also interpretable, providing users with a clear understanding of how decisions are made. For instance, in scenarios like credit scoring, an XAI system wouldn't just reject a loan application with a vague response like "Application denied". Instead, it would offer a clear, transparent reason, such as "Your credit score is below the required threshold".
XAI works by breaking down the AI decision-making process into understandable steps. It shows the factors that were considered in the decision and explains how each factor influenced the final outcome. This level of transparency is crucial in areas like finance, healthcare, and legal decisions, where the reasoning behind an AI’s decision can have significant implications.
How XAI Differs from White Box AI
It’s easy to conflate Explainable AI with White Box AI, but while they are related, they are not the same. White Box AI refers broadly to AI systems that are transparent and interpretable, meaning their internal workings are accessible and can be understood by humans. On the other hand, XAI goes a step further by ensuring that AI decisions are not only accessible but also user-friendly and easy to interpret for non-experts.
In other words, while all XAI is white box AI, not all white box AI systems are designed with the same level of user-centric transparency. XAI focuses on making the decision-making process more intuitive and easily explainable, which is particularly useful in industries where understanding and trust in AI are crucial.
AI Transparency Tools
To support transparency and interpretability in AI, a range of AI transparency tools have been developed. These tools help users understand how AI models process data and arrive at decisions. For example, in a loan application scenario, transparency tools could highlight the key factors—such as income level and loan history—that influenced the AI's decision to approve or deny a loan. By showing which data points were most critical, these tools make the decision-making process more transparent, enabling users to better trust and evaluate the AI’s predictions.
Such transparency tools are increasingly vital in sectors like banking, healthcare, and even hiring, where understanding AI-driven outcomes is essential for accountability and compliance with ethical standards.
Ethical AI Practices
As AI continues to influence various aspects of society, the demand for ethical AI practices has grown. Ethical AI focuses on ensuring that AI systems are designed, developed, and deployed in ways that are transparent, fair, and unbiased.
For example, regular audits of AI hiring algorithms can help detect and correct potential biases that may favor certain candidates based on gender, ethnicity, or age. By implementing routine checks, organizations can ensure that their AI systems make fair decisions, avoiding discrimination and promoting diversity.
Ethical AI is not just about ensuring fairness in decision-making but also about protecting user privacy, securing data, and fostering trust between AI systems and their users. With increased regulation, such as the European Union’s AI Act, organizations are being held accountable for ensuring their AI systems comply with ethical guidelines, and the use of explainable and transparent AI is becoming an industry standard.
The Future of Black Box AI
The future of black box AI is uncertain, largely due to the many questions and challenges it presents. While black box AI systems are highly effective in certain applications, their lack of transparency makes it difficult for users to fully understand or trust how decisions are made. This has led to growing calls for more accountability, regulation, and explainability in AI development.
As black box AI continues to evolve, researchers and policymakers are working to find ways to address its limitations. The ultimate goal is to strike a balance between the power of these complex models and the transparency required for ethical and safe use. This evolution is likely to involve significant regulatory action and technological advances, particularly in fields like healthcare, finance, and education, where AI decisions can have profound implications.
Regulatory Movements in the U.S. and EU
Both the United States and the European Union (EU) have already taken steps to regulate black box AI and reduce its risks. These measures are aimed at increasing transparency, protecting users, and ensuring the ethical use of AI technologies.
In the U.S., key developments include:
- The Consumer Financial Protection Bureau (CFPB) has mandated that financial companies using black box AI credit models must provide consumers with clear reasons if their loan applications are denied. This ensures a minimum level of transparency in the financial sector.
- A 2023 Executive Order from the Biden administration requires AI developers to share critical testing data with the government, with the National Institute of Standards and Technology (NIST) tasked with developing safety standards for AI systems.
- The release of the SAFE Innovation Framework, led by Senate Majority Leader Chuck Schumer, is designed to encourage debate on AI research and ensure that black box AI models are both safe and used for positive purposes.
In the European Union, regulatory actions are even more robust:
- The AI Act, passed in 2023, is the world's first comprehensive law focused on regulating black box AI systems. It establishes guidelines on the risks and privacy concerns associated with AI while promoting trust and accountability.
- The EU has also started addressing the use of AI in key areas such as education, healthcare, criminal justice, and even military applications, with particular attention to controversial technologies like facial recognition in public spaces.
These regulatory efforts underscore the global push to ensure that AI, especially black box models, are used responsibly and transparently.
The Rise of Explainable AI and Hybrid Models
As black box AI becomes more widely adopted, efforts are also underway to make these systems more interpretable. The development of Explainable AI (XAI), which provides insights into how AI systems make decisions, is playing a key role in this transformation. XAI techniques can break down complex models into understandable components, allowing users to see which factors contributed to a decision. This is especially important in high-risk sectors like healthcare, where AI systems are already diagnosing diseases and recommending treatments.
Looking ahead, we are likely to see the emergence of hybrid AI models that combine the accuracy and complexity of black box AI with the transparency of more interpretable models. These hybrid systems will aim to retain the power of black box AI while offering explainability for critical decision-making processes. For instance, a medical AI system might use a complex neural network to diagnose diseases but also provide clear explanations of its reasoning, ensuring doctors can trust its conclusions.
Building Trust Through Transparency
One of the key challenges for the future of black box AI is building trust. As AI becomes more embedded in daily life, users will need to feel confident that these systems are making decisions fairly and safely. This is particularly true in industries like finance and criminal justice, where a lack of transparency could lead to biased or unethical outcomes.
Efforts to make black box AI more transparent will likely include better tools for interpreting models, as well as new regulations to enforce transparency and accountability. For example, in healthcare, regulators may require that AI systems provide clear explanations for their diagnoses, ensuring that patients and healthcare providers can trust the AI's recommendations.
The Path Forward: Balancing Power and Interpretability
The future of black box AI will depend on finding a balance between powerful algorithms and clear, interpretable results. As researchers develop more advanced methods to observe and explain these systems, black box AI models may become easier to understand without sacrificing their predictive capabilities.
Ongoing innovation in AI, coupled with thoughtful regulation, will help ensure that black box AI systems are both powerful and ethical. By enhancing transparency, explainability, and accountability, the future of black box AI can be one where users feel confident in trusting AI for decisions that impact their lives.
In conclusion, while black box AI remains a powerful tool, its future will be shaped by efforts to reduce its opacity and increase trust through transparency and regulation. Whether through regulatory frameworks or technological advancements like hybrid models and XAI, the future of AI will likely prioritize both accuracy and accountability, ensuring that AI can be harnessed safely and effectively in a wide range of applications
Please note that Plisio also offers you:
Create Crypto Invoices in 2 Clicks and Accept Crypto Donations
14 integrations
- BigCommerce
- Ecwid
- Magento
- Opencart
- osCommerce
- PrestaShop
- VirtueMart
- WHMCS
- WooCommerce
- X-Cart
- Zen Cart
- Easy Digital Downloads
- Blesta
- ShopWare
- Botble
- Zender
- XenForo
- CS-Cart
10 libraries for the most popular programming languages
- PHP Library
- Python Library
- React Library
- Vue Library
- NodeJS Library
- Android sdk Library
- C#
- Ruby
- Java
- Kotlin
19 cryptocurrencies and 12 blockchains
- Bitcoin (BTC)
- Ethereum (ETH)
- Ethereum Classic (ETC)
- Tron (TRX)
- Litecoin (LTC)
- Dash (DASH)
- DogeCoin (DOGE)
- Zcash (ZEC)
- Bitcoin Cash (BCH)
- Tether (USDT) ERC20 and TRX20 and BEP-20
- Shiba INU (SHIB) ERC-20
- BitTorrent (BTT) TRC-20
- Binance Coin(BNB) BEP-20
- Binance USD (BUSD) BEP-20
- USD Coin (USDC) ERC-20
- TrueUSD (TUSD) ERC-20
- Monero (XMR)